By Toni Manzano (Aizon), Mario Stassen (Stassen Pharmaconsult BV), William Whitford (DPS Group) and AIO Team
In February of 2022, the efforts of Xavier Health were assumed by the AFDO/RAPS Healthcare Products Collaborative. Because of the important work done before this transition, the Collaborative has chosen to retain documents that have Xavier branding and continue to provide them to the communities through this website. If you have questions, please contact us at info@healthcareproducts.org.
Having data of quality is the first step in the Artificial Intelligence (AI)/ Machine Learning (ML) journey to get reliable models and valuable AI/ML outputs. There are many issues regarding data quality that can affect the design of the algorithm as well its implementation and operation. These concerns include gaps in time-series, different criteria to manage outliers, merging discrete variables and events with time-series, bias during the string categorization process, and forced interpolation. This type of variety in data is due to a few sources, including variability in governance procedures.
The good news regarding these challenges is that they have a mathematical solution. Data with these types of discrepancies can be rectified using good practices in data selection, pre-processing and repair from modern data science.
Challenge:
There are data issues that require a higher level of control and additional care regarding the full process of data management, and this is especially true when the results derived from this data have a significant impact on the patient’s health. Particular concern with the data required by developing AI/ML solutions in pharmaceutical operations comes from a few different sources. Many of the earlier uses of data generated by multiple and disparate assays, instrumentation and locations had short-lived and local uses− and therefore didn’t require current standards of continuity, integrity and labelling. There are first-hand reports of pilot AI/ML projects encountering unexpected delays due to data access, aggregation, organization and repair. Significantly, the number of cites with data integrity issues in warning letters published by the FDA is still too high to be considered as a superfluous problem in the pharmaceutical industry. Furthermore, the most repeated issue regarding data integrity is to work with incomplete or missing records (appears in the 67% of the warning letters), although, from a regulatory perspective, there are other findings with this level of criticality− such as inappropriate manual integration of data or destruction of original Good Manufacturing Practice (GMP) records (see the graphic below).
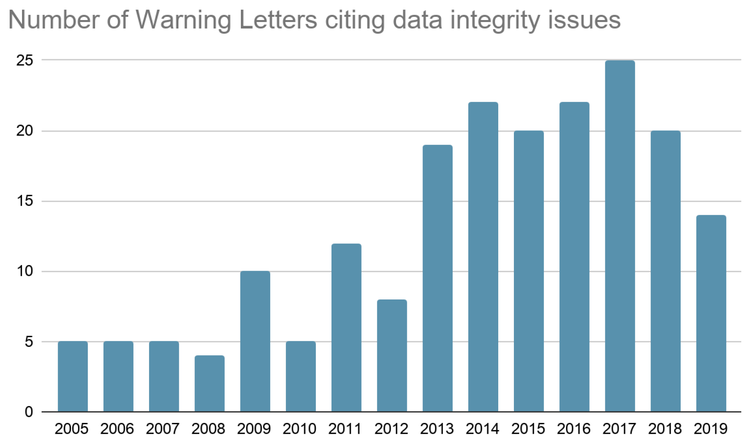
Large quantities of good data are the raw material for reliable AI, ML and Deep Learning(DL) results. The use of information that contains the defects mentioned above cannot lead to the acceptable results in terms of predictions, automatic classifications, pattern recognition, clustering or recommendation.
Solution:
Demand for data integrity presents yet another benefit from the extensive use of AI, ML and DL in pharmaceuticals today. The data governance required in the AI field that has been traditionally linked to the FAIR principles (findable, accessible, interoperable and reusable) can be considered as a good ally in the original ALCOA principles of attributable, legible, contemporaneous, original, accurate plus the newer parameters, complete, consistent, enduring, and available. Therefore, ensuring data integrity becomes a requirement in AI practices, with new data curation activities being part of the science behind the process that transforms data into knowledge.
Do you face problems with the adequacy of your data?
Invaluable Resource:
A whitepaper, Data Quality for AI in Healthcare, published February 2021, might help. It was developed under the leadership of the Xavier Health program at Xavier University in partnership with industry professionals, as a planned output from the 2019 AI Summit. This document addresses data quality through the entire data lifecycle, including characterizing the data to be acquired, potential bias in the data, data selection, cleaning and manipulation, and storage. Additional topics such as data streaming, risk management, etc., are also discussed. You can find a free copy at this link.
This post is part of a series called AI in Pharma Adoption:
- Part 1: Why is AI Not Broadly Adopted in the Lifescience Industries (Pharma, Biologics and Medical Devices)?
- Part 2: “Good AI Begins With Good Data”
- Part 3: AI is Transforming Manufacturing—The Hows and Whys
- Part 4: Three Real Use Cases of AI Implementation in Pharma – NOT Science Fiction!
- Part 5: AI Value in Complex, Unstructured and Multiparametric Data