By Toni Manzano (Aizon), Mario Stassen (Stassen Pharmaconsult BV), William Whitford (DPS Group) and AIO Team
In February of 2022, the efforts of Xavier Health were assumed by the AFDO/RAPS Healthcare Products Collaborative. Because of the important work done before this transition, the Collaborative has chosen to retain documents that have Xavier branding and continue to provide them to the communities through this website. If you have questions, please contact us at info@healthcareproducts.org.
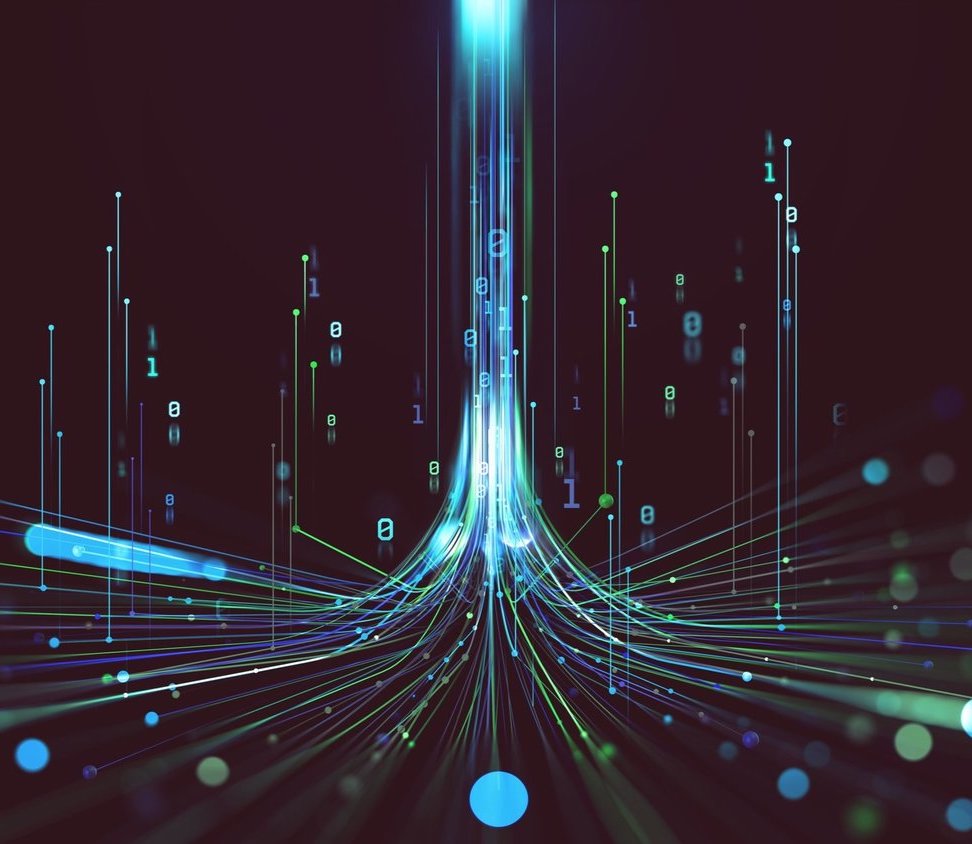
AI is a combination of statistics, math, algorithms and software able to establish links between the complexity of reality and the emergence of specific behaviors created by the intricate relationships of the studied units. The human body is maybe one of the most complex systems where myriads of entities are in harmony by means of a delicate balance based on innumerable chemical, physical and biological interactions. AI is the best science to reproduce and manage issues raised from acute or chronic deficiencies in any of the countless metabolic systems in the body.
The latest AI technologies support the use of comprehensively monitored experimentation or studies, review of past operations, and even review of the scientific literature to provide solutions in a number of disciplines in medicine. Even more, with advancements in AI technology, there is a renewed interest in such sub-topics as the treatment of rare diseases. Some have observed that the field of artificial intelligence is hampered by fear on one hand, and excessive optimism on the other. It is an imperative for AI professionals to educate corporate leaders in the true potential of, and investments required for AI maturity in practice.
Some data-heavy projects appear to be a rather easy task for existing AI applications, but are actually very hard or even not yet possible. Take the current version of CAPTCHA as an example. It might seem surprising that even early, readily available AI-impowered machine vision tools can’t easily find all images containing a bicycle or stoplight.
Then, there are projects that appear to be enormously difficult, such as those demanding analysis using much unlabeled or unstructured data, or data sets from multiple, disparate analytics. Yet, it is sometimes possible for such projects to yield easily to standard AI approaches. Many digitally enabled medical or pharmaceutical manufacturing project designs demand the use of data from multiple analytics and probes, or new high-throughput and high-density analytics, requiring highly-multiple single parametric or multi-parametric analysis. AI (including machine learning and deep learning) has become a powerful and robust tool in providing reliable solutions from multiple sources and types of data-rich analytics of various relationships. The hierarchical algorithms of deep learning provide a unique nonlinear approach to analysis in such applications. They support identifying specific, identified relationships in large amounts of data representing very different aspects of a system, or from data which are poorly structured or poorly labeled.
Linearity is not a property usually inherent to the biological systems and even less when biotech processes used to produce drugs require multiple phases, equipment and forced conditions to accelerate the growth rate, yield of the final biologic output harvested in the cell cultures or when SME interact with the systems to lead the process to the expected results. Algorithms, such as the Iterative Dichotomizer, bring amazing results in biotech applying entropy aspects in the algorithmic calculations. Therefore, the units of information gained iteration by iteration are accumulated establishing the entropic constraints along the process. This algorithm and its enhancements (like the C4.5) are good allies in biotech systems to identify dynamic evolution of complex structures.
Complex, Unstructured and Multiparametric Data
Analysis of any single type of omics data provides knowledge of some reactive processes. But, since biological processes are so interrelated, understanding any single system in the context of the others is important. For example, we understand that while regulation of protein expression is influenced by upregulated mRNA, it alone may or may not enhance its target protein expression. This is because of such factors as the influence of the protein’s associated metabolites and miRNAs (a class of non-coding RNAs that play important roles in regulating gene expression) ability to silence or degrade mRNAs. A more holistic approach, elucidating the interconnectivity and interdependence of many omics, requires a far more complete and comprehensive integration of multi-omics data.
Many on-line databases are appearing to accommodate multi-omics data. Integrated multi-omic analysis on large populations provides a path of information flow from one omics data to other omics data thereby providing power for analysis supporting therapeutic development [1]. Such types of analysis, provided in a timely fashion, can also directly support the field of individualized or precision medicine. Comprehensive integration of such multi-omic systems and non-omic data is challenging because of the size, heterogeneity, and complexity of relationship between such data sets. AI is uniquely qualified to generate any number of models supporting a systems approach to such analysis.
Systems Biology
Relatedly, it has been observed that the 20th century style of reductionism has provided much understanding in, and labeling of, a systems parts−but is unable to complete our understanding of the system, or interpret any components or subprocesses that are currently unstructured. Systems, or network, biology is a new approach to understanding the complex interactions of the molecules in life, using an integrative approach to such complex systems expressing synergistic or emergent behavior. It accomplishes this by modeling these complex biological interactions by comprehensively compiling information from interdisciplinary fields. For example, AI empowered systems biology supports mapping of the molecular relationships in normal and abnormal phenotype. This promises to result in a more explicit and deterministic model, providing more predictive, preventive, and personalized medicine.
Multi-omic Analysis in Research
There are many new technologies defining new approaches to the design of a disease prevention or therapy. Many depend upon understand of the dynamic relationship of such biological entities as genes, proteins, mRNAs, miRNAs, metabolites, as examined at a global scale. The human body contains almost 20,000 proteins and protein-coding genes, 30,000 mRNAs, 2,000 miRNAs and over 100,000 metabolites. Not only is analyses of large numbers of bio-entities becoming possible, but so is a comprehensive understanding of their interplay. We are even seeing the possibility of such modeling to be applied to creating profiles for identified subpopulations or even individuals.
It is impossible to functionally integrate the volume and diversity of multi-omics data by classical methods to produce this more holistic understanding [2]. This approach relies upon advanced tools and methods to provide such a multi-platform based omic data analysis and interpretation [3]. The tools employed have included network, Bayesian, correlation-based, and other multivariate methods− and are now being empowered by AI.
Digital Pathology
Digital pathology is being enabled by commercialized systems now entering the market. These products apply AI and machine learning to improve diagnoses and other medical functions for variety of diseases. AI empowered solutions promise to combine the expertise of modern pathology with algorithms developed to both improve the quality of micrographs, as well as improve the accuracy of their interpretation.
Computerized tomography (CT) is an imaging technique supporting digital pathology. It uses special x-ray equipment, and sometimes a contrast material, to produce multiple images slices through the body.
Positron emission tomography (PET) is an imaging technique that uses radiotracers to visualize and measure changes in physiological activities including blood flow, regional chemical composition, and absorption. The standard uptake values (SUV) it can produce, based upon static images, allows PET to quantify the uptake of tracer in a tissue. However, the distribution of tracer in the body is a dynamic process influenced by factors specific to each tissue. Therefor SUV PET cannot always indicate accurate final tracer distribution.
AI is now allowing the combination of PET with computed tomography (CT) or magnetic resonance imaging (MRI). This image fusion or co-registration allows doctors to interpret composite images built upon information from two different types of exams. This leads to more precise information and a more exact diagnosis. AI empowered PET/CT can perform two exams simultaneously providing images of, for example, SUV, metabolic glucose rate (Ki), and distribution volume (DV) (Figure 1).
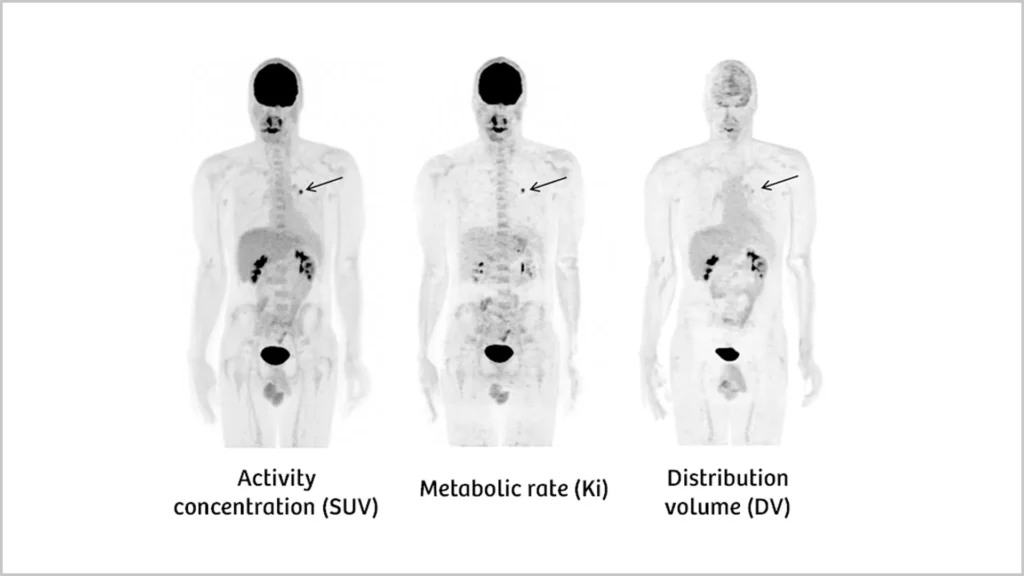
Spatial biology is an emerging field providing understanding of cells and tissues within their native 2D or 3D context. Based upon the newest genomics and transcriptomics methods, it employs both protein and nucleic acid labeling and monitoring. The number and complexity of data generated demands powerful software solutions to provide coordinated analysis of multiple biomarkers and visualization of the tissue’s spatial architecture.
Medical Diagnostics
Enormous volumes of personal health data are employed by providers to deliver appropriate care and successful care. But producing a diagnosis and therapy from these disparate pieces of data is difficult because of the patient data structure. Patient data can exist in structured, unstructured and hybrid sets. Structured data exists in a clearly defined tabular format. Unstructured data is not organized in any kind of pre-defined manner. Semi-structured data is a hybrid of the two types: it does not exist in a common tabular structure, but it does use some sort of consistent labeling or organization to denote fields and record relationships. One example of semi-structured data is notes from a medical professional. They can include valuable observations and information that, while not in a standard computer data format, do reside within a discernable grammatical structure that AI can interpret.
Biopharmaceutical Operations Process Prediction
Digital twins are a virtual model of an operation, such as the cell culture, and operate by synthesizing data from a number of measured physical, chemical and biological values representing the reactor data’s dynamic (and dynamical) relationships. AI can be valuable in both the construction of the model, as well as in its application toward a number of applications. For example, data from the past and current state of both the cells within a reactor, as well as the control parameters, can be used by such a model to support the processes, analyses, development, prediction and control.
Conclusion
Extracting and making sense of data in medicine and pharma is still an issue, and AI can be the answer to it. The manual analysis of large datasets to identify potential new drug candidates is particularly time-consuming. Much data is available, but this data should be put into a form that can be analyzed. Natural Language Processing and Deep Learning, two key aspects of artificial intelligence, are solutions to meet this objective.
REFERENCES FOR FURTHER READING
- Kaur, N., Bhattacharya, S. & Butte, A.J. Big Data in Nephrology. Nat Rev Nephrol (2021). https://doi.org/10.1038/s41581-021-00439-x.
- Tolani, P., Gupta,S., Yadav, K., Aggarwal, S. Amit Kumar Yadav. A. K. (2021). Big data, integrative omics and network biology, Advances in Protein Chemistry and Structural Biology, Academic Press, ISSN 1876-1623, In Press. https://doi.org/10.1016/bs.apcsb.2021.03.006, https://www.sciencedirect.com/science/article/pii/S1876162321000407.
- Huang, S., Chaudhary, K., Garmire, L. X. (2017). More Is Better: Recent Progress in Multi-Omics Data Integration Methods, Frontiers in Genetics, 8, (84), 1-12. https://www.frontiersin.org/article/10.3389/fgene.2017.00084
This post is part of a series called AI in Pharma Adoption:
- Part 1: Why is AI Not Broadly Adopted in the Lifescience Industries (Pharma, Biologics and Medical Devices)?
- Part 2: “Good AI Begins With Good Data”
- Part 3: AI is Transforming Manufacturing—The Hows and Whys
- Part 4: Three Real Use Cases of AI Implementation in Pharma – NOT Science Fiction!
- Part 5: AI Value in Complex, Unstructured and Multiparametric Data